FlowerAura Implements Data Lake Solution and Recommendations Engine for Actionable, Real-time Insights Into Customer's Behavior
FlowerAura wanted a cloud solution provider with state-of-the-art capabilities, it chose AWS as its Cloud vendor and Searce expertise for setting up a secure, durable, and highly scalable storage for its data lake. Along with it, to be more customer centric and to deliver immediate value to your end user or business, Recommendation engine was added to have a more personalized experience.
- FlowerAura delivers fresh cut flowers handpicked by expert florists in more than 220 cities
- They have a strong affiliate network and channel stores to cater to an ever growing client base.
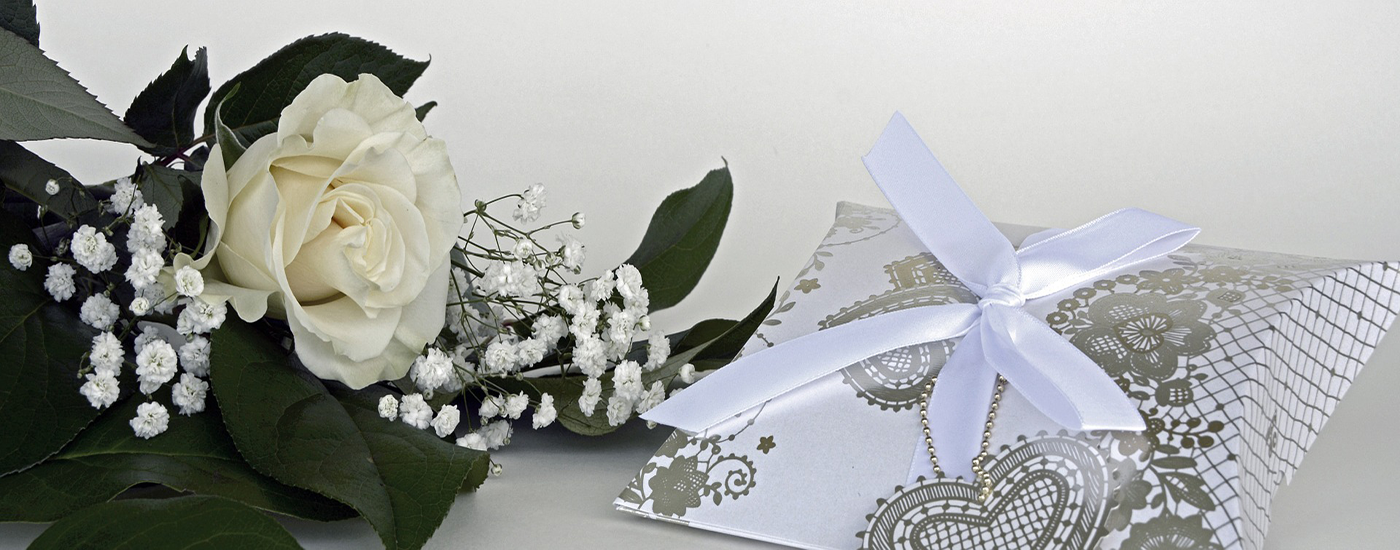
FlowerAura’s Need to implement Data Lake and Recommendation Engine
FlowerAura has thousands of items for sale in its online store. In addition, more than 250 million people visit the company’s websites every year. With a massive number of product searches and transactions taking place in a huge online store, FlowerAura constantly generates enormous amounts of data. FlowerAura had data sources for ClickStream data, Relational Data and Log data in their infrastructure on which they wanted to implement a data lake and recommendation engine.
- Enhance its BI capabilities, For cost-effectiveness and scalability on the way to store and analyze its data. Leading to move its analytical data to the cloud for easier scalability
- Perform ETL on all their unstructured and semi-structured data
- Implement a recommendation engine that would help the customers/visitors to make a better decision while purchasing
FlowerAura’s Journey from Data pool to Data Lake
Flower Aura wanted a secure, durable, and highly scalable storage for its analytical data, Searce Expertise used S3, to build a data lake that collected real-time data from the existing data sources and used AWS Glue which performed ETL on the collected data.
The transformed data was then trained using Sagemaker which provided recommendations to the customer as per the browsing and purchasing history.
For the journey from Data pool to data lake, the workflow designed to help the company included:
- Automation in consolidating all the data sources into a single central repository
- A VPC on AWS with Public and Private subnets, NAT Gateway, security group rules, etc.
- Site to Site VPN established with the on-premise data center(s) to securely pull data from their existing database system
- AWS Kinesis data streams used for all the real time data from the source
- The ETL jobs will be configured to persist raw data on S3 along with the transformed data to target AWS Sagemaker
- Sagemaker was used to train the data as per the data received from S3 bucket for predictive analysis and recommendation to customers
- The data from Redshift is sent to AWS Quicksight for creating and publishing interactive dashboards
- It also leads to greater customer satisfaction, higher retention of existing customers, and lower advertising cost
Noticeable outcomes of using AWS Quicksight
- Reduced Manual Intervention - Automation in consolidating all the data sources into a single central repository
- Easy Insights - Reducing complexity and time to value to deeper insights
- Central Data Store - Creating a central repository for all of the data sources reduced the effort of collating data from various sources and converting schema for different types of datasets to relate and make meaningful insights from existing data. Also this has reduced the licensing cost as no additional Database is required to keep different types of data
- Better and Faster Predictions - The recommendation engine presented users with choices regarding items from their list of available items
- Better Customer Retention - Not getting the right product in time encourages customers to shift to competitors. Now presenting with the right items at the right time leads to greater customer satisfaction, higher retention of existing customers, and lower advertising costs
more case studies