Searce Helps Colive To Predict Inventory Requirements And Forecast Revenue Possibilities
Colive relied completely on a manual rule based formula for calculations which was not based on any intelligence or past learning experience or data. Results with this approach were fixed and completely based on the manual input factors. They wanted to replace this model with a more intelligent model.
- Colive offers rental homes to 20,000+ single professionals & young couples
- All homes are fully serviced & professionally managed while offering flexible & affordable options suitable for urban living.
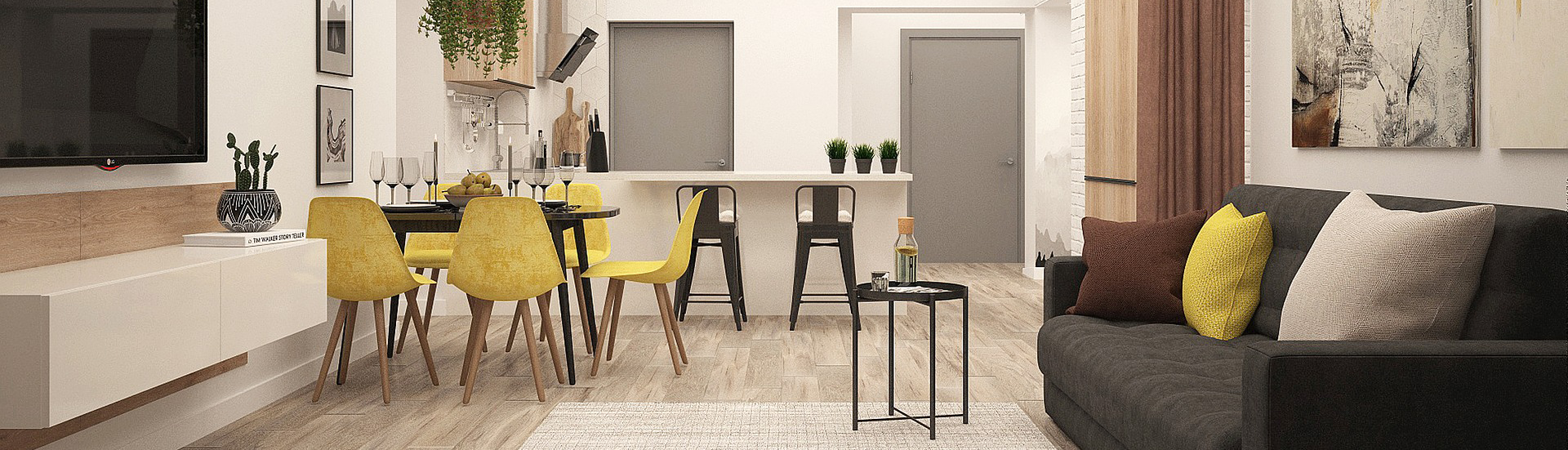
Colive’s Targeted Business Needs to Automate the Prediction Cycle
- Training Cycle: Colive wanted to make the training and improvement easier which in turn would reduce the span of overall manual revenue calculation.
- Expansion Challenge: With the increase in the number of customers the data collected due to user activity was increasing on a daily basis. They wanted to automate the prediction cycle for the tenure of each property being rented.
Searce Solution to Predict the Traction of Rental Stays
A team of data scientists from Searce had a detailed discussion to understand the entire flow of data collection & data type. Searce collaborated closely with Colive’s Data Analysts/business teams and created a training data which was clean & flawless. Monthly, fortnightly or quarterly basis predictions were made by this model which recommended the caretakers to arrange the resources and prepare the facilities.
The data used in this Forecast were of following types:
- Target data set (Check in date, Location of stay)
- Related time series data set (Time frame)
- Item metadata - Supporting data sets for prediction (Marital status, Age, Gender, etc.)
A collaboration of these input data points helped us perform the prediction with high accuracy. This was helpful for the management teams to predict the traction of the stay during a particular time frame, which had a great impact on the preparation of stay facilities.
The AWS Approach using an Agile Model of Project Delivery
- Automated training pipeline: Amazon Forecast reduced a lot of manual overhead of training and improving the data prediction model. This also reduced the training cycle.
- Improved results: An intelligent Machine Learning model replaced an old rule based system which resulted in better prediction accuracy.
- Future-ready: The current deployment was provisioned utilizing all the scalable services on AWS. This helped Colive to achieve the desired flexibility with the increasing number of users and increasing data size.
- Extension: More complicated calculations and predictions could be now conducted by implementing this model. Predictions like – possible stay period, expected growth in the number of guests, change in revenue, etc. were predicted with higher accuracy.
more case studies